Ventus Therapeutics
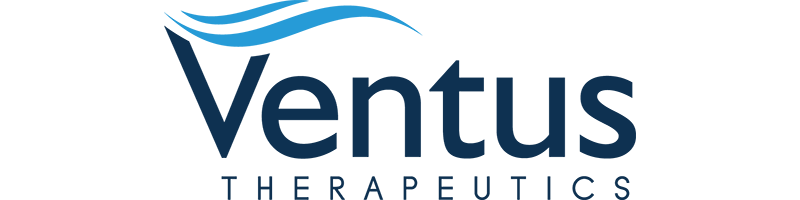
Marcelo Bigal
CEO
Ventus Therapeutics is a clinical-stage biopharmaceutical company deploying leading-edge technologies to discover new drug candidates, which can then be used to develop new medicines.
President and CEO, Marcelo Bigal, explains how AI has enabled the company to bring three medicines to clinical trials in only five years and shares his view on the need to think more deeply today about what it means to be human.
Can you tell us about Ventus Therapeutics?
Ventus Therapeutics develops small-molecule medicines for difficult-to-drug proteins associated with very important diseases by taking advantage of proprietary and novel technologies including AI and machine learning.
So, what does that mean and why is it significant?
Medicines come in many forms. They can be antibodies, genetic modifications, stem cell therapies. The medicines that people know and trust the most are what we call small molecules. These are things like the vitamins we might take in the morning, tablets prescribed by our doctors, the most common injections that we receive.
Small molecules work to improve health by being able to modify disease-causing proteins. The protein is large, and the molecule is small, and if there is a pocket on the protein that is the right shape, the small molecule can bind to the protein and disrupt it.
When we started the company five years ago, there was a sense that whatever proteins could be drugged using small molecules had been drugged already. And many of the proteins that caused significant diseases didn't offer a visible pocket to be drugged.
So, while the industry was moving from tried-and-tested to more experimental modes of medicine, we believed that we could still solve the problem with small molecules through using proprietary and novel technologies.
And where does AI sit in this mix of proprietary and novel technologies?
We use AI algorithms in three main ways. But first, you need to understand the problem with identifying small molecules that could be used to drug proteins.
Let's say that we want to be able to drug a particular protein. And that protein is inside a cell. A cell is water and salt. The protein is not static. It is changing shape and adopting new conformations.
So, the first part of the problem is how to identify druggable pockets in a protein that is continuously changing shape.
Think about if you were trying to understand a ballerina dancing but you only have a photo. You can describe the ballerina, and talk about height, weight, hair color, etc. but you cannot describe the dance.
So, we developed our first AI/ML algorithm that models the dance of the proteins. This allows us to define how many conformations the protein assumes in its ballet. Once we define all these conformations using this algorithm, we identify if any of the conformations has a pocket that could potentially be drugged by a small molecule.
The second problem is identifying the small molecules that could potentially drug those pockets.
You have your protein doing its dance inside the cell in water that’s also moving. And the pocket that we want to drug is full of water that is also moving. We developed our second AI/ML algorithm to map the movement and behavior of the water inside the pocket. We call this the Hydrocophore®.
And, using a third AI component, we digitally extract the Hydrocophore® and use it as a blueprint to screen massive virtual libraries of chemical matter. When we have identified all the virtual hits, the AI/ML part of the process ends and we bring it back to the real world and synthesize the molecules in a chemical lab. We test them and optimize them and, if successful, we start developing drugs, typically using chemistry and biology.
Are there other aspects of your work where AI may be applied?
Currently we use AI/ML to visualize the dance of the protein, to map and extract the Hydrocophore®, and to screen the virtual libraries of potential small molecules that could be candidates for drug development.
This is an incredible model that is way beyond the limitations of previous trial-and-error approaches. But it is still limited because we need to extract the pocket on a case-by-case basis.
The pocket of protein A is very different from the pocket of protein B, which means the Hydrocophore® blueprint for each will be very different. And whatever I learn for protein A doesn't teach me anything for protein B.
So, what if we could use machine learning to extrapolate all the different shapes of pockets and extract Hydrocophore® for all proteins to define optimal small molecules for those pockets? The initial problem is that you don’t have the required training data. You can teach machine learning to recognize cats online because there are so many pictures of cats online in the first place. The training data set has to be bigger than the problem that you're solving in order for you to deduce the answer.
When you realize that the permutations that small molecules can make are 1063 – that’s a one with 63 zeros – it’s apparent that you can’t use machine learning to solve this problem simply by training on the structures of the small molecules, as many are trying to do, as there is no way to have a large enough training set to develop foundational models that can be used across different targets.
However, with our method, we don’t rely on the permutations that small molecules can assume. We rely on the physics of water.
Water is H2O, however, it has many permutations for how it behaves in pockets, but way less than the 1063 possible permutations of small molecules. These permutations of water are defined by the laws of physics. And the laws of physics are finite. You can create training data sets to model water in the pocket if you know how to do it.
So, the machine will learn all the permutations that water can assume and train itself to create a universal method for identifying chemical molecules that fit with difficult-to-bind proteins. And that includes molecules that don’t yet exist.
I think that this will be profoundly disruptive. Think about a world where, instead of taking 15 years to develop a medication, it takes six. I say six because the duration of the clinical trials still remains the same. But, within those trials, instead of having a 20% probability of success, you have an 80% probability of success because of the increased ML-driven rationality driving decisions at multiple stages, from selecting chemical compounds to predicting safety and efficacy.
What this will mean in terms of benefit for patients and in terms of costs is a profoundly disruptive leap that we believe machine learning can bring to drug development.
Do you see any wider ethical concerns in relation to the use of AI?
Let me start by saying that I am a huge fan of AI. I love the idea that it will help us develop medications that can treat myself, my wife, my kids, my friends. So, I am massively in favor. But I do have concerns about how little thought we, as a society, have given about what we want to achieve with AI.
A drug for a disease? That’s a good thing. Predicting weather changes so we have better farming and avoid starvation? Awesome. Or preventing weather changes in the first place? Even better. Improved traffic movement? That's a great thing.
But would it be a good thing to get AI to replace journalists? AI will be able to do this interview eventually. And AI will be able to answer on my behalf. Is that good?
Would it be good to use AI to make coffee? To replace baristas? How useful would it be to make a large fraction of society unnecessary – not needed? And the answer for me is the human touch. I would rather get coffee from a barista who interacts with me than from a robot. I would rather go to a flesh-and-bones human doctor.
If we want to continue to be relevant, we have to acknowledge that machines will be smarter than us in 100% of things. And being relevant comes from consistently maintaining what makes us human. And what makes us humans, I believe, is that we have reason and that we are social by nature.
I am absolutely convinced that it's impossible for us to keep pace with machines, which means we truly need to redefine humanity and be responsible in how we use technology in relation to that humanity.
I had a professor when I was in medical school who said that one of the problems with science is that for every 10 people doing science, there was only one thinking science. And historically scientists have done some terrible things, and a lot of terrible things were done in the name of science. Too many people doing science, very few people thinking science.
And the same may be true for AI. We have a lot of people doing AI. Some of them for very good reasons – to benefit all – and some for less good reasons but not enough people really thinking AI.
Meet the dream makers
Hear directly from the visionaries leading the SoftBank Group’s portfolio companies that are shaping the future of medicine and health.